Computer-Assisted Coding: The Future of Medical Billing Efficiency
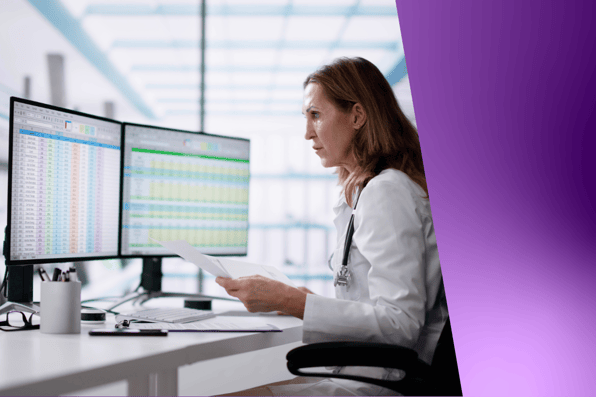
Coding systems have been around for several decades, but are notoriously time-consuming and hard to master. Computer-assisted coding (CAC) is a newer process of using technology to analyze and interpret information and find and assign the best code.
It’s sometimes called automated medical coding and is one way medical coders are adapting to the sheer volume of data in healthcare. While digital tools and software help simplify data recording and sharing, it’s also tedious and expensive to filter and review so much input. CAC aims to revamp the coding workflow, making it easier and faster to summarize information and assign accurate codes with lower costs.
Also, more modern tools using AI in medical coding are offering better ways to improve efficiency. Newer AI technologies learn from data and get smarter over time, offering better performance. For insight into the potential future of medical coding, read on.
How CAC Works
AI and automation are complex technologies that involve many different solutions. In short, CAC uses AI to read and interpret clinical notes and suggest codes based on that data.
The technology is part of a billing solution that integrates with your electronic health records (EHR) and usually involves one of two main types of AI in medical coding:
- Deep learning: A type of machine learning that uses layers of networks to process large data sets.
- Natural language processing (NLP): A combination of machine learning and statistical modeling that finds patterns, interprets meaning, and creates human-like responses.
NLP tools automatically read the notes for key phrases, procedures, and diagnoses, and check them against codes and ICD sets. The AI matches the data to the most likely codes, and you select the best one, validate it, and add it to the claim.
Automated medical coding might also suggest additional codes that apply based on information, which may help you find revenue you might have missed. As you select codes and validate information, the program will learn from your input and make connections with data and codes in the future.
Benefits of Using Computer-Assisted Coding
If you struggle with a billing backlog or a high denial rate, CAC software may be the answer. These tools speed up your workflow, boosting productivity, lowering errors, and enhancing cash flow.
Higher Productivity
Manual medical coding is demanding, during which staff pore over notes and review code books or other documents to find the correct class. The average coding professional codes and processes 60 cases per day, which means it can take months to move through pending cases.
Automated CAC workflows speed up the review process by scanning and summarizing text for you, leading to a more efficient workflow and higher productivity. In fact, one study found that CAC software boosted productivity by 20% when a coder reviewed and validated the suggestions.
Fewer Coding Errors and Better Cash Flow
Manual coding is also error-prone and accuracy varies widely, depending on the complexity, the diagnosis or service, and the coder. The industry accuracy ranges from 50% to 98% — with a median of 80% — which leads to claims issues.
Adding automation tech, however, reduces mistakes and improves billing and claim accuracy. Several studies have looked at how well AI in medical coding can accurately assign codes and found that NLP models have a coding accuracy of 91% in older model versions and 99% in newer ones.
The Purpose of AI and Machine Learning in CAC
Automated coding is changing the way large systems operate by improving billing practices and increasing capacity within your systems.
Lessens Staff Workload
AI and machine learning add flexibility and intelligence to the system, increasing your system’s capacity. Technology serves to simplify and speed up tasks, and AI in medical coding does just that.
It’s designed to extract meaning and assign codes and does it at a faster rate than humans can. You recapture time lost in manual coding, freeing staff to manage and review more complex cases.
For instance, a hospital system studied AI software using real-time EHR data and found that AI lowered coding time by an average of 40% and improved corrections on EMR mistakes. The software was three times better at finding missing codes than manual coders, making it valuable for prediction and review.
Sharpens Billing Practices
Almost 20% of claims in the healthcare industry face denial, and roughly 60% of those are never corrected and resubmitted. Plus, it costs practices an average of $25 per claim to resubmit and hospitals an average of $181.
However, AI can increase coding accuracy, which improves claims submissions, boosts productivity, and strengthens billing practices. Claims approved in the first round lead to better cash flow and improve financial stability.
Potential Pitfalls of Automated Coding
AI isn’t perfect, even with all its benefits. Data quality and human oversight are some of the more pressing issues that you’ll need to consider.
Inconsistent Notes Degrade Results
NLP models need good data to produce quality results. These models learn over time, so poor clinical documents may lead to unreliable summaries and code predictions. You can curb this problem by making sure clinical records are detailed and include the proper information with the relevant documents to improve accuracy.
AI Shouldn't Run Unchecked
Data suggest that automated coding performs better when humans are in the loop and can review, correct, and validate AI predictions. A study on CAC compared the software to a combined use of software with oversight and found that software alone was less precise and had a lower recall.
In addition, coders and billing staff need to understand how the software arrived at its coding selections. Reviewing the suggested codes and comparing them against keywords or phrases within the notes can confirm accuracy. And lower the risk of errors, bias, or noncompliance.